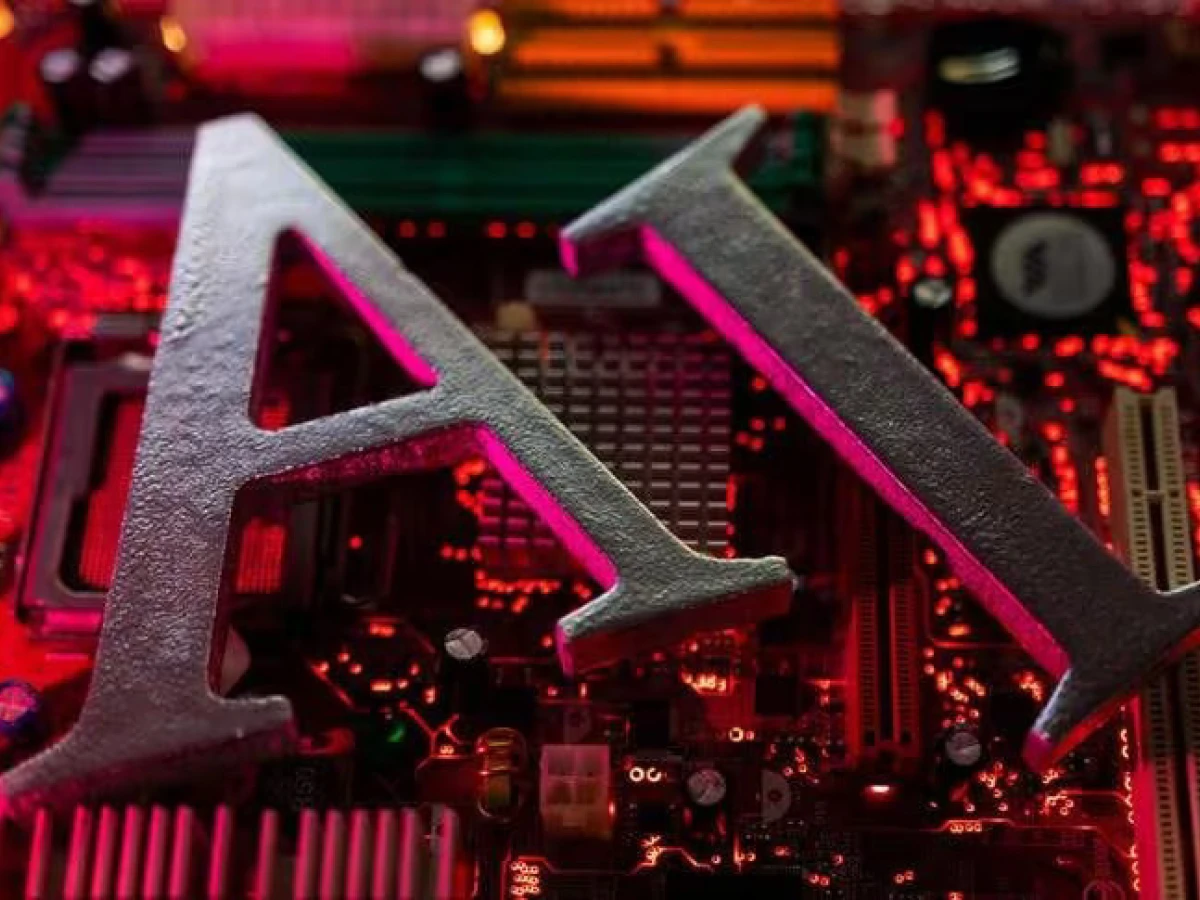
The economics of AI points to value of good data
Companies which own useful, specialised data will be the biggest winners from AI.
LONDON, June 28 (Reuters Breakingviews) - Nvidia (NVDA.O), opens new tab briefly became the most valuable company in the world last week after shares in the leading supplier of chips and networking infrastructure used to train artificial intelligence models nearly tripled since January. Yet the AI revolution has so far proved far from a one-way bet: most of the stocks in a host of AI-focused indices and funds, opens new tab are down this year.
Even the $3.1 trillion Nvidia has been a wild ride. In the three trading sessions following its historic peak, it lost more than $400 billion in market value. The week before it had added $360 billion. Over the past three years, its share price volatility has been five times higher than the S&P 500 Index.
These epic gyrations reflect investor uncertainty over the economics of AI. The achievements and promise of self-teaching computers are obvious. How much the technology costs and who will pay for it is less clear. For investors seeking to navigate this treacherous landscape, it is important to start with the technological advance on which the current AI revolution depends.
The stunning applications that have sparked the AI boom seem very different at first sight. In March 2016, Google DeepMind’s AlphaGo program wowed the world when it beat all-time great Lee Sedol at the two-person board game. In November 2020, the company’s AlphaFold algorithm cracked one of the grand challenges in life sciences by predicting, opens new tab the protein structures novel combinations of amino acids will form. Two years later, OpenAI seemed to be doing something completely different again when it launched a natural language chatbot capable of ad-libbing Shakespearean verse.
Yet these landmarks all stem from the same innovation: a dramatic improvement in the accuracy of computerised predictive modelling, as AI pioneer Rich Sutton explained in a 2019 blog post, opens new tab. For decades, researchers trained computers to play games and solve problems by encoding hard-won human knowledge. They effectively tried to mimic our ability to reason. But these attempts were eventually bested by a far less complicated approach. Naive learning algorithms proved consistently superior when they were fuelled with sufficient computing power and fed with sufficient data. “Building in our discoveries,” Sutton concluded, “only makes it harder to see how the discovering process can be done.”
This lesson is familiar. In the best-selling 2015 book “Superforecasting: The Art and Science of Prediction”, Canadian psychologist Philip Tetlock and his co-author Dan Gardner explained that the same agnostic method is a winner for humans too. In prediction tournaments, methodical and open-minded amateurs systematically outperform. Common sense plus the willingness to absorb a lot of data is more effective than deep domain knowledge and specialist expertise. Today’s frontier AI models essentially automate the superforecasters’ approach.
This simple recipe – learning algorithms plus computing power plus data – produces prodigious predictive results. It also provides a guide to where the long-term value in AI lies.
Start with the algorithms. The non-profit research institute Epoch AI estimates, opens new tab that between 2012 and 2023, the computing power required to reach a set performance threshold has halved approximately every eight months. Such are the cost efficiencies captured by recent innovation in neural networks.
Yet the long-term value in these algorithms is much trickier to pin down. Digital code is vulnerable to imitation and theft. The pace of future innovation is difficult to predict. The human talent currently sitting in AI labs owned by tech giants can easily walk out of the door.
The second ingredient – brute computing power – is a simpler proposition. According to Epoch AI, it has generated the lion’s share of the gains in the performance of AI models. The soaring market values of the largest providers of cloud computing – Alphabet (GOOGL.O), opens new tab, Amazon (AMZN.O), opens new tab and Microsoft (MSFT.O), opens new tab – suggests stock markets have discounted many of the gains. Yet a new manifesto, opens new tab for AI investing by former OpenAI staffer Leo Aschenbrenner, opens new tab argues investors should not be deterred.
Since model performance is tightly linked to the volume of chips and electricity deployed, he urges investors to “trust the trendlines” and “count the OOMs” – a reference to the orders of magnitude by which performance has accelerated year-by-year – in order to project capital spending.
Doing so yields requirements so enormous it puts even the most bullish industry projections in the shade. In December, opens new tab, Nvidia rival AMD (AMD.O), opens new tab forecast that the market for AI chips would reach $400 billion by 2027. Trusting the trendlines implies that AI investment will hit $3 trillion just a year later, while the first datacentre cluster costing $1 trillion will open two years after that. Count the OOMs and it appears that computer hardware, not software, is now eating the world, opens new tab.
Yet there is a flaw in this reasoning. The first two ingredients of AI – algorithms and computing – are worth nothing without the third: data. What is more, the better the data, the less valuable processing power becomes.
This fact has been easy to overlook. The most prominent AI applications are general-purpose chatbots which have been trained on sprawling hauls, opens new tab of unvetted text harvested from the web. They preferred quantity to quality, with computing power left to compensate. Training OpenAI’s ChatGPT 4 was estimated, opens new tab by Morgan Stanley to have involved at least 10,000 graphics chips crunching well over 9.5 petabytes of text, opens new tab. That compromise determined the result: remarkably lifelike interlocutors which are prone to incorrigible hallucinations and increasingly at risk of costly litigation for copyright infringement.
Special-purpose applications of AI have a lower profile but demonstrate where the future is more likely to lie. Nobel Prize-winning scientist Venki Ramakrishnan said Google DeepMind’s AlphaFold model solved “a fifty-year grand challenge in biology, opens new tab”. Just as remarkable is the fact that it required the equivalent of fewer than 200 graphics chips, opens new tab. That was possible because it was trained on a painstakingly curated database, opens new tab of 170,000 protein samples. High-quality data therefore not only radically improves the effectiveness of AI models, but also the economics of the technology.
Companies which own useful, specialised data will be the biggest winners from AI. It’s true that richly valued tech giants such as Google owner Alphabet and Amazon dominate some of that space too. Yet much less glamorous – and more reasonably priced – banks, utilities, healthcare providers and retailers are sitting on AI goldmines too.
Datasets, not datacentres, are where the real value of the AI revolution lies.